Alberto Gutiérrez has recently presented his Master’s Thesis within the scope of the GenObIA project, this development is entitled “Multi-level classifier for the identification of individuals at risk of developing overweight”. In this work a classifier system based on artificial intelligence and machine learning techniques has been developed. The system consists of a novel multilevel classification mechanism in which up to three different classifiers are combined. These have been selected from a set of 14 supervised classification algorithms through a previous cross-validation process. The classifier works by tiering different classification algorithms and setting thresholds for the acceptance of each algorithm’s response. The classification algorithms provide both the group they assign and the probability that the classification is correct. If the probability provided by the first algorithm is higher than this threshold, the classifier’s decision is considered correct, otherwise a classification is requested to the next level algorithm and so on.
This multilevel classifier has been specifically designed to fit the Genobia-CM project data, although its design allows it to be applied to any other problem using the appropriate input data format, which is the usual one in classification problems. Genobia is a project involving a consortium of 20 institutions, hospitals and companies, financed by the European Social Fund and the Community of Madrid. The project seeks to design, using artificial intelligence, predictive algorithms for the identification of people at risk of developing overweight, obesity and their associated pathologies. In this work we have used a database with 1179 individuals provided by the Consortium in which information on lifestyle habits and adherence to the Mediterranean diet is collected. The multilevel classifier has been implemented as a predictive and classification algorithm for the risk of being overweight, adapting it to the data provided where the number of cases of obesity is very small. Our proposal reduces the number of false negatives (cases of people who are overweight classified as not being overweight), which is fundamental to the problem in question, since, being a matter of public health, this implies reducing the number of erroneous or omitted clinical actions.
The results obtained are around 80% accuracy rate and our system is perfectly prepared to accept the data provided by the consortium in the future. These data will include genetic information of each individual and we expect that it will also include a larger number of cases. In addition, other types of classifiers based on decision trees have been performed, as well as an exhaustive analysis of the variables, their influence on the models, redundancies and a study of the sensitivity of the models to them.
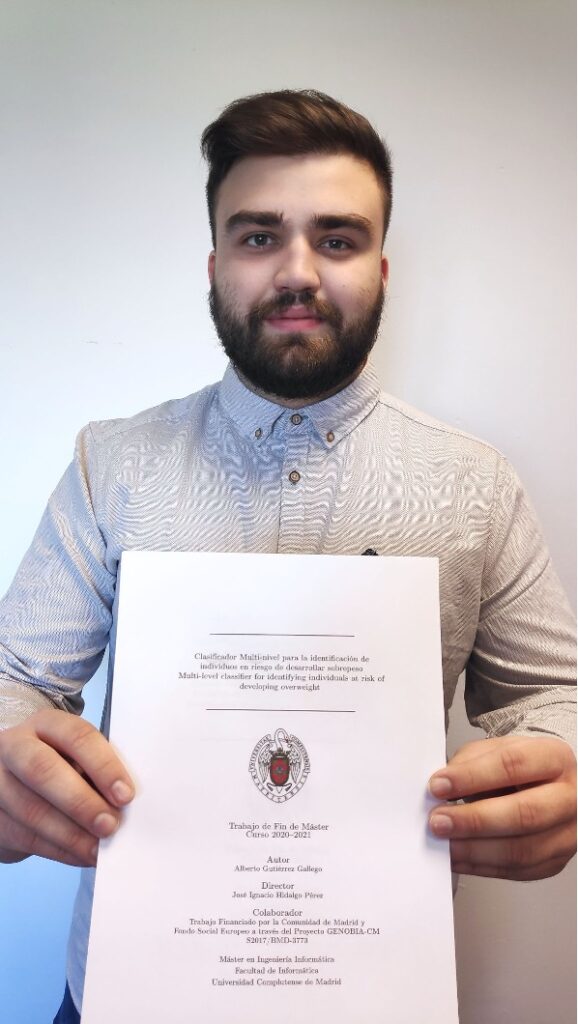